Data management and data governance. They may sound like similar data handling practices with the same end goal of leveraging the full potential of organizational data, but they are distinct concepts with individual goals.
Let us dig deeper into their similarities and differences to understand their individual and combined roles in ensuring the integrity and usefulness of your organization’s data.
What is data management?
Data management refers to a set of processes and tools for ingesting, organizing, storing, cataloging, transforming, and sharing organizational data to maintain data availability, security, and reliability. It’s an umbrella term that encompasses a wide range of data-handling activities, including data governance, quality management, integration, analysis, and protection.
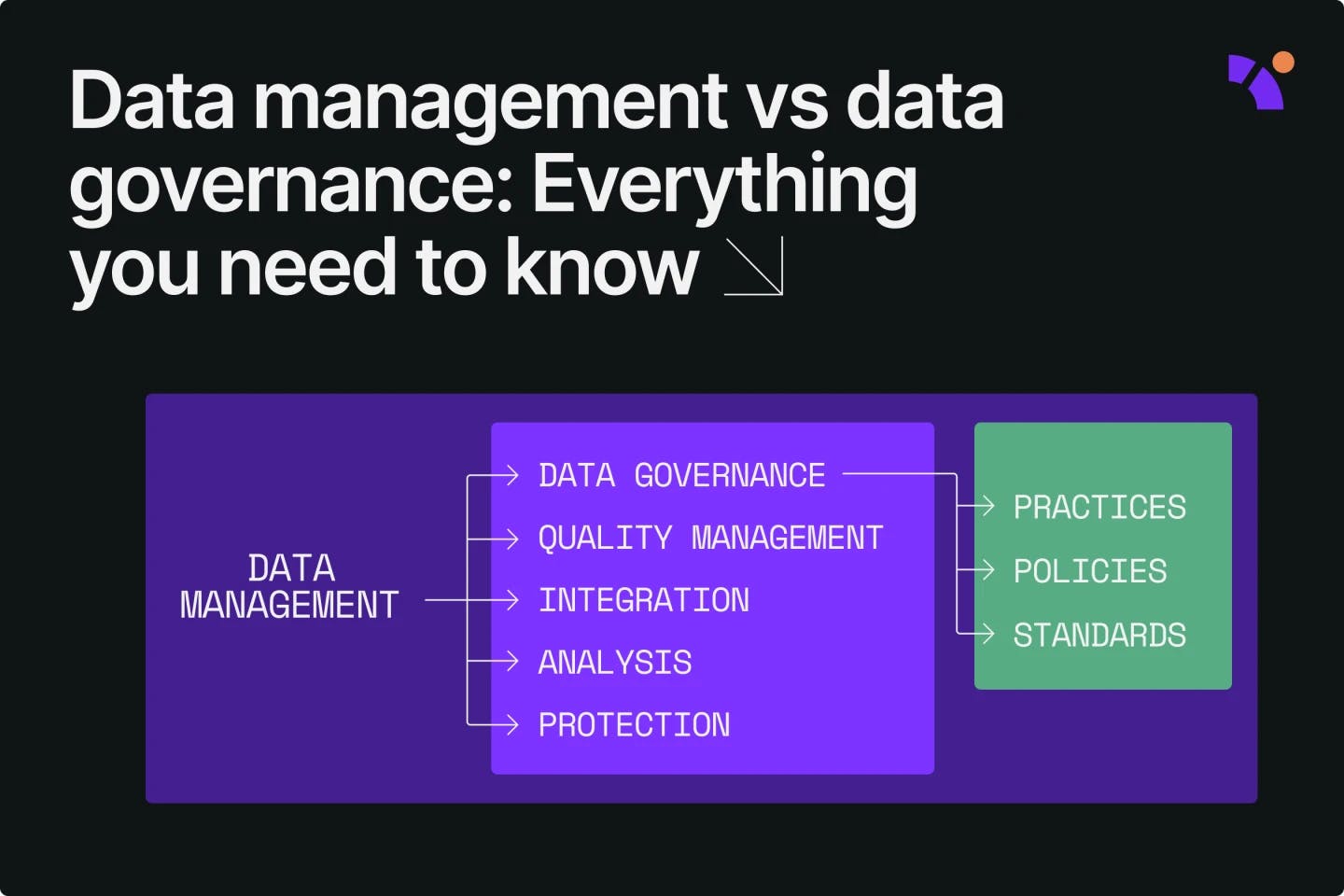
The goal of data management is to ensure that the organization’s data is accurate, reliable, and valuable enough to aid in decision-making. It continues to be essential for organizations to generate maximum value from their rapidly growing data.
A few key data management processes include:
- Defining and dedicating tools for data acquisition, storage, transformation, modeling, quality, integration, security, and governance
- Monitoring all data operations from collection to analytics
- Ensuring compliance with governance regulations
- Implementing a data governance framework
Here are some of the questions that data management addresses:
- How can data be consolidated from multiple sources?
- How can data be collected and stored to ensure accuracy and consistency?
- What tools can be used for data storage, transformation, and analysis?
- How can data be cleaned and transformed to ensure quality and integrity?
- How can we ensure timely and secure data availability for different users and systems?
- How can data be protected against unauthorized access, alteration, and deletion?
- How can data sharing be facilitated between teams?
What is data governance?
Data governance is a set of practices, policies, and standards applied to the collection, storage, use, protection, preservation, and deletion of data. It ensures availability, usability, integrity, and security and serves as the guideline for implementing data management tasks.
Data governance achieves this by doing the following:
- Establishing and enforcing data policies
- Creating governance structures
- Assigning and confirming data owners
- Controlling access to data
- Setting and recording data metrics
- Documenting and monitoring processes
Here are some of the questions that data governance addresses:
- How and from where is data collected?
- How is data used to support regulatory compliance?
- How is data lineage tracked and understood?
- How is sensitive information stored and protected?
- How is access assigned to data assets? Who has access to what data, and who is allowed to modify data?
- What metrics can we use to ensure high data quality?
Data management vs. data governance: What’s the difference?
The following are the key differences between data management and data governance:
Purpose and processes
Data governance is about establishing the procedures and policies for how the organization will use data. Some of the processes involved in data governance include:
- Establishing data quality guidelines
- Setting up data lineage to ensure traceability
- Monitoring management practices to ensure compliance
In comparison, data management spans the day-to-day processes of data usage by implementing the standards set by data governance. Examples of processes involved in data management include:
- Data integration for a unified data view
- Implementing security controls for data protection
- Data archiving to prevent loss
Tools and technology
Effective data management heavily relies on an efficient stack of tools associated with data operations. These include tools for data processing, exploration, transformation, and more.
In contrast, effective data governance encompasses strategic and planning processes that require tools for documenting data ownership and access policies and integrating them with all data assets for compliance checks. Examples of such data governance tools include data lineage, data contracts, catalogs, dictionaries, and glossaries.
Roles and responsibilities
Data management involves tasks such as data ingestion, storage, and distribution. These are typically performed by data engineers and architects. In contrast, data governance involves decision-making responsibilities such as determining data policies and assigning roles and responsibilities for data management. Data owners and domain business experts typically share data governance responsibilities.
Focus
Data management focuses on the technical aspects of data, such as its format and structure, while data governance focuses on the business and compliance aspects, such as how it supports organizational goals and complies with legal and regulatory requirements.
Despite their conceptual differences, data governance and data management aim to achieve the same goal: managing data as a valuable asset. Both involve establishing policies and procedures for data quality, security, and accessibility and are concerned with compliance with relevant laws and regulations. Both also include identifying and assigning roles and responsibilities to ensure data accuracy, completeness, and consistency.
Additionally, both aim to provide data access and usage to the right people at the right time. They require regular monitoring and oversight to ensure that data management practices are consistent with data governance policies and procedures. Both ultimately aim to support data-driven decision-making and improve the organization’s overall performance.
Use cases for data management and data governance
Data management use cases
Data management is necessary for any organization that collects, stores, and analyzes large amounts of data. Some use cases for data management work include:
- Data warehousing: This is the collection and storage of data in a central location, such as a data warehouse or data lake. Additional tasks in this use case involve data organization, indexing, and accessibility for analysis and reporting.
- Data transformation: The conversion of data into a format that is aligned with the requirements of its target systems. It involves mapping data to predefined structures and cleaning and transforming it to maintain consistency across systems. The focus of transformation is to ensure that data is in the relevant format for its intended use.
- Data modeling: This is the process of creating a data model that depicts individual data elements and the relationships between them. It involves designing the structure of databases and data systems so that it aligns with real-world data representations and organizational requirements. Data management ensures effective data modeling to provide optimized data for analysis and decision-making.
- Data analytics: This involves using data management processes like data mining, machine learning, and business intelligence techniques to extract insights and knowledge from data.
- Data quality control: This includes detecting and correcting data errors such as duplicate records and data inconsistencies. It also includes keeping check of data freshness to avoid outdated data usage. Data management for quality control ensures data accuracy and usability for high-value analysis and decision-making.
Data governance use cases
Data governance is vital for any organization where operations rely on the accuracy and security of its data. Organizations under strict data laws and regulations can benefit from data governance to ensure compliance. For example, healthcare organizations need to comply with HIPAA regulations. Some specific data governance use cases include:
- Setting data governance metrics: Data governance sets metrics like data quality, usage, and security as measurements used to track and evaluate the effectiveness of data governance practices.
- Creating a data governance framework: This involves putting together a framework of all the policies, processes, standards, and tools used to manage and govern data. It includes guidelines for how teams and individuals should handle and use data and who is responsible for it.
- Assembling a data governance board: The people involved in overseeing and implementing a data governance program within an organization play a key role. Data governance ensures the responsible allocation of administrative roles to representatives from different departments, such as IT, legal, compliance, and business units.
When do you need data management and data governance?
Data governance is engraved in data management as a blueprint for the guidelines required to execute all data management practices. So, it should come as no surprise that, in addition to their isolated benefits, they can work together to enhance how your organization deals with data to extract the most value from it.
In the following use cases, you will find data governance and data management enabling one another to complete a data management lifecycle:
- Ensuring compliance: Data management processes, such as data masking and archiving, ensure that data complies with regulations and laws. At the same time, data governance policies and procedures provide oversight and guidance on compliance requirements and reporting.
- Implementing data security: Data management practices, such as encryption and access controls, ensure sensitive data is protected from unauthorized access. Data governance practices, in this case, provide oversight and guidance on data security policies and procedures.
- Improving data quality: In this scenario, data management processes, such as data cleaning and validation, ensure that the data is accurate and consistent. Simultaneously, data governance policies and procedures provide oversight and guidance on collecting, storing, and using data to maintain high quality.
Make DataOps efficient with data management and data governance
Without data management, data governance is merely the documentation of rules and standards. Similarly, without data governance, data management is just a bunch of haphazard data operations implemented without any guidelines.
To improve the efficiency of your data operations, not only do you need data management and governance to work together, but you also need the right tools in your data stack to automate your management practices. Y42 is such a tool.
Category
In this article
Share this article