Data lineage is the practice of tracking data flow within an organization. It involves identifying and documenting the origin of data, how it is transformed and used, and where it is stored.
In practice, data lineage is frequently represented visually using diagrams or flowcharts that depict the movement of data from its source to its destination. These representations may include information on the many processes, systems, and tools used to transform and store the data, as well as intermediary phases and data storage locations along the way.
A data lineage for a simple data pipeline might include the following components: the source of the data, the data storage locations, the data transformation processes, and the data consumption processes or tools that use the data.
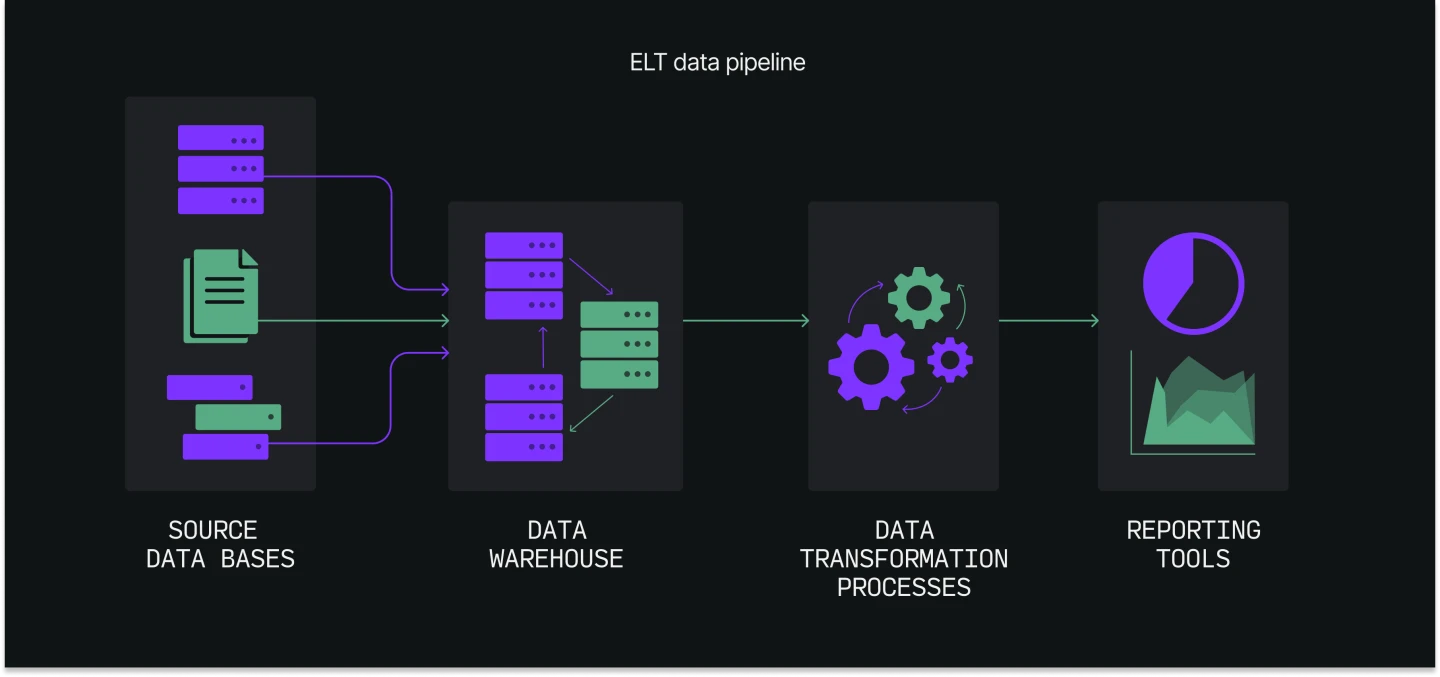
Data lineage diagrams can be complex and may include many different data sources, transformations, and destinations, depending on the size and complexity of the organization’s data ecosystem.
Why is data lineage important?
Data lineage enables you to understand the history and context of your data. This is critical in the following cases:
- Debugging: Debugging is much easier when the data flow across your systems is well-known. Data lineage helps determine the root cause of any issues or errors that arise.
- Auditing: A well-documented data lifecycle and history make it much easier to demonstrate compliance with regulatory requirements or industry standards.
- Data governance: Careful data governance allows you to avoid problems with the data’s quality, privacy, security, integrity, and compliance.
- Data quality: You may detect and manage data quality issues more quickly if you have a clear view and understanding of your data’s origin and history. Data quality is vital for ensuring that data-driven decision-making is relevant and trustworthy.
Without data lineage, data professionals may struggle to understand the flow of data within their systems and identify any issues or errors that occur. Moreover, a poorly documented data lineage can cause issues with data integrity, compliance, governance, and quality.
High-quality data lineage provides a clear and visual representation of the data’s journey through the data pipeline. In this way, it can facilitate communication between different teams and departments or shorten the time it takes for new hires to start contributing.
How does data lineage work?
Data lineage works by tracking data movement, including the data’s origin, transformation, storage location, and use. This process typically involves the following steps:
- Identify the data sources: The first step in data lineage is to identify all the data sources, including databases, file systems, and web APIs.
- Document the data transformation processes: Data lineage also involves documenting the various processes and tools used to transform the data, such as filtering, aggregation, cleansing, or enrichment.
- Track the data storage locations: The next step is to track the various locations in which the data is stored after it is transformed, such as data warehouses, file systems, and cloud storage buckets.
- Document the data consumption processes: You will need to document the different processes and tools that use the data, such as reporting tools, machine learning models, and dashboards.
- Visualize the data lineage: Finally, data lineage is visualized by employing diagrams or flowcharts to depict the data flow from source to destination.
Data lineage is an ongoing process, and organizations should regularly maintain their data lineage documentation to ensure that it is accurate and up to date.
Data lineage vs. data classification
Data classification is the process of organizing data into categories based on its content, sensitivity, or other characteristics.
Data classification is often used to help organizations manage and protect their data assets by identifying and labeling data based on its level of sensitivity or importance. For instance, an organization might classify data as public, internal, or confidential depending on the required level of access and protection.
Data classification and data lineage are related, but they serve different purposes. Data lineage enables you to understand data’s history and context, while data classification helps you identify and manage data according to its content and sensitivity. Together, these processes help you better understand, control, and protect data assets.
Data lineage vs. data provenance
Data provenance refers to the history of a specific piece of data, including its origin, ownership, and any transformations it has undergone.
Data provenance is used to establish the authenticity and reliability of data and to trace its history to understand how it was created and used.
Data provenance and data lineage are related, as both involve tracking the history and movement of data. However, while data lineage looks at the overall movement of data within an organization, data provenance focuses on the specific history of a particular piece of data.
What are the common data lineage techniques?
Several common techniques are used to implement data lineage, including the following:
- Visual representation: Data lineage is often represented visually. These visualizations include information about the data sources, transformation processes, storage locations, and consumption processes.
- Metadata tracking: Data lineage can also be tracked using metadata — data that describes and contextualizes other data assets. A collection of metadata makes up what is known as a data catalog (the inventory of all the data an organization has).
- Data dictionaries: Data dictionaries are documents that provide information about the data within an organization, including definitions, attributes, and relationships.
- Process flow diagrams: Process flow diagrams are visual representations of business processes that show the workflow within an organization.
- Technical specifications: Technical specifications are detailed documents that describe the technical requirements and specifications of systems, tools, and processes. These documents provide information about how data is transformed and used within different systems and processes.
Applying these techniques together will result in a clear and efficient data lineage.
Data lineage use cases
There are many potential use cases for data lineage. Here are some examples:
- Data-driven decision-making: Data lineage is mandatory for businesses that rely on data-driven decision-making, as it can help you understand the context and reliability of the data being used.
- Data security: Data lineage can help you understand how sensitive data is being used and where it is stored. This knowledge is vital for data security.
- Operational efficiency: System bottlenecks or inefficiencies can be identified by analyzing the flow of data, which can help improve operational efficiency.
- Customer insights: A thorough analysis of the data origin and history may provide insights into customer behavior and preferences. These insights can be useful for marketing.
From a broader perspective, data lineage can also be utilized for:
- Research and development: Data lineage can be useful for research and development, as it provides the history and context of data used in research projects.
- Supply chain management: Data lineage can be used to trace the flow of goods and materials through a supply chain, helping teams understand the origin and history of the products they sell.
Gain a holistic overview of your data pipelines with Y42
Data lineage is an essential aspect of DataOps that enables you to understand the history and context of data. By tracking and documenting data’s flow and transformation, you can improve data quality, governance, and management and facilitate collaboration and communication within your organization. However, maintaining high-quality data lineage can be a challenging task, especially in large and complex data ecosystems.
Y42, the Modern DataOps Cloud, includes features such as data lineage tracking and data lineage visualization, which help data teams establish and maintain a clear and comprehensive overview of all their data.
A dedicated tab allows you to access the data lineage of all the pipelines you have built on Y42. You can also examine the provenance of any asset in the data pipeline at any time or location in the webapp. This feature allows you to quickly and reliably determine where the data comes from and where it is used. The following example shows how the Y42 platform allows for simple navigation through a data pipeline.
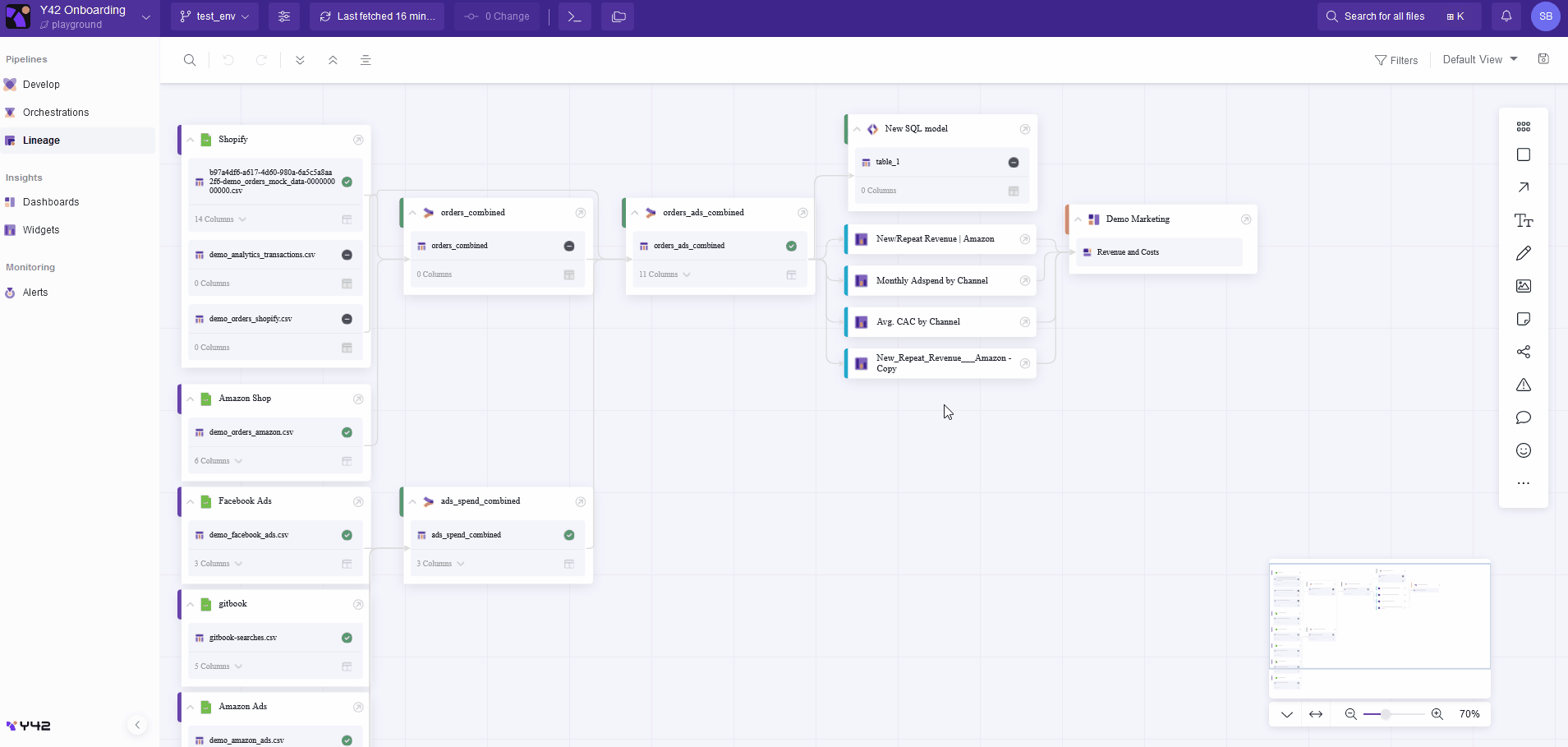
Data lineage is also strongly enhanced thanks to the data catalog, as it contains editable metadata. Y42 then allows you to filter pipeline views on asset types (e.g., integration, SQL models, or dashboard), job status (e.g., ready, pending, or canceled), or asset status (e.g., draft, deprecated, or verified). Tags can be added to pipeline assets, too. Tags refer to metadata assigned to assets in order to classify and categorize them. With tags, it is possible to segment and filter data ecosystem views according to specific business functions or departments (such as marketing, finance, and HR), allowing for more targeted and relevant data lineage investigation.
Category
In this article
Share this article