Why do data teams continue to struggle with the same data problems despite technological advancements and increased investment in data-driven solutions? According to a leading consulting firm’s recent survey, out of 97% of organizations that invest in data initiatives, only 26.5% report having successfully created a data-driven organization.
So, are data teams failing to evolve with technology? Or have the challenges data teams face evolved to become unmanageable? There’s no simple answer. Data teams today must navigate complex data ecosystems and a constantly changing technological landscape. They also have to deal with the intricacies of data governance, data quality, and data integration. Data teams face organizational and cultural barriers — such as siloed data and resistance to change — that can prevent them from collaborating effectively and delivering value to their organizations.
“While businesses are generating more data than they know, data teams are scuffling to provide value and sustain momentum in operations.” — Matheus Dellagnelo, CEO at Indicium
Data problems in modern organizations span way beyond their data teams’ control. In this article, we’ll dig deeper into some of the more common challenges data teams face and discuss actions your team can take to overcome them.
5 common challenges data teams face
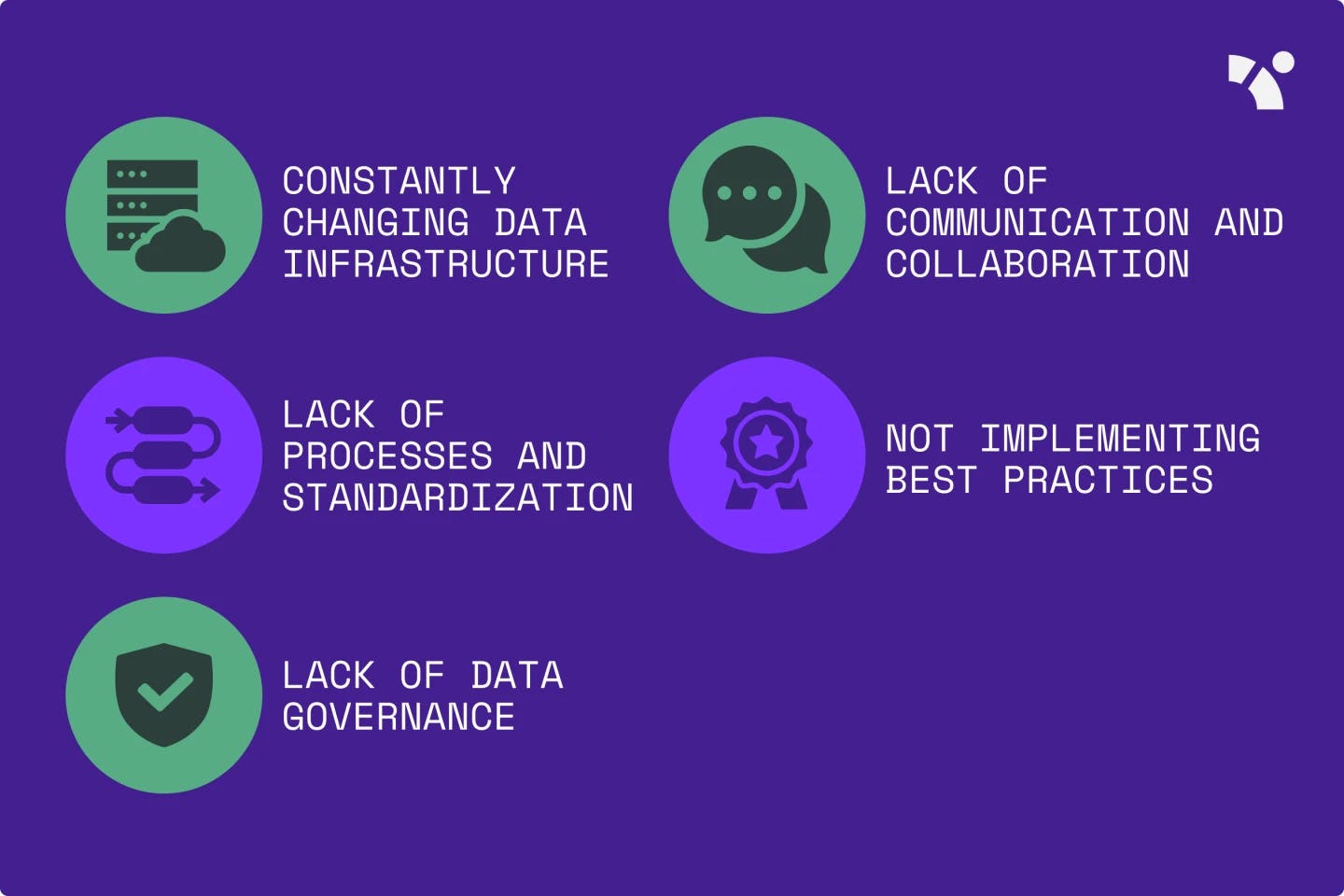
A data infrastructure that constantly changes
One of the most common challenges data teams face is a constantly changing data infrastructure. This is particularly true for organizations with high turnover rates or that regularly hire new developers.
When new developers come in, they may want to implement their preferred tools or methodologies, which requires significant changes to the existing infrastructure. This can be frustrating for data teams as it can slow their progress, lead to inconsistencies in data management, and make it difficult to maintain data quality. As a consequence, delays in data analysis, reporting, and other data-related tasks are common, which impact the team’s overall productivity.
As an extreme example, two developers may prefer to use different databases, resulting in data being stored in multiple locations. This makes it difficult to retrieve and analyze data and adds to the challenge of maintaining data quality and consistency.
An effective way to address this challenge is to regularly review and incrementally update your data infrastructure to ensure that it remains relevant. This might involve identifying areas where new systems may be beneficial and where existing infrastructure may need to be improved or updated. For example, you might decide to upgrade from an on-prem storage solution to a cloud-based data warehouse. This is similar to the approach software developers adopt: They regularly update libraries (i.e., small parts of their code) as they go out of date.
Lack of communication and collaboration between data producers and data consumers
Another common challenge is a lack of communication and collaboration between data producers and data consumers. A typical scenario arises when business teams change the tools they use (which serve as the data source for data teams) without informing the data engineers. This stops the flow of data and results in data quality issues.
The communication gap between data team members also increases when data professionals lack business context and when business users lack technical context. When data professionals fail to understand business context and are constantly misaligned with business users, it leads to slow turnaround of data products and multiple iteration cycles. Meanwhile, when business users lack technical context, it leads to unreasonable requests and friction with the data team.
For example, imagine a business team update the field names in Salesforce without informing the data team. The data team continue using the old field name, and the pipeline breaks. This is similar to what would happen if you didn’t properly connect two separate parts of an oil pipeline: you would get an oil leak. In this case, it’s a data leak. This leads to data quality issues, such as data inconsistencies across teams and data loss. What’s more, the data team wouldn’t be able to perform quality checks on the updated data.
Data contracts are a possible solution. They are agreements between data producers and data consumers that define the data format, quality, and other relevant details that help to ensure everyone is on the same page about the data, its intended use, and how it should be consumed.
To set up a data contract, start by identifying the data elements at play in a collaborative effort between your data producers and consumers. Next, you need to document the contract and make it available to all stakeholders. Once the data contract is in place, you need to regularly monitor compliance to identify issues early and ensure that the data is accurate, consistent, and up-to-date.
Lack of processes and standardization
Another problem that data teams commonly struggle with is a lack of proper processes and standardization. Without proper processes in place, maintaining documentation, data quality, and consistency across the organization can be chaotic.
“Data standards affect what one can learn from data, regardless of whether the data has been shared.” — Data Standardization by Gal & Rubinfeld
For instance, when there is no clear process for defining metrics, different team members may use different definitions, leading to confusion and incorrect analysis. Additionally, when new models are built, team members may create new logic for defining metrics, further contributing to inconsistencies and limited data usage.
However, while standardization is important, it’s also essential to ensure that processes are not overcomplicated, as this can lead to team members ignoring them and finding workarounds. You need to strike a balance between simplicity and having enough structure to ensure consistency and efficiency.
Using a data catalog to define metrics can help you implement clear and concise processes. Data catalogs provide a centralized location for storing and managing metadata about data assets, including data definitions, owners, quality, and usage. By implementing a data catalog, you can ensure that everyone is using the same definitions for key metrics and that data assets are consistently documented and understood.
Not implementing best practices
As data teams grow in size, organizations can face several challenges when best practices are not implemented in a standardized way.
One common problem that this can cause is difficulty in maintaining a clear line of communication within the team. For example, a data scientist needs to collaborate with a data engineer. However, not clearly communicating their requirements to the data engineer could lead to unfulfilled data infrastructure or processing needs. This communication gap increases when the processes for how data should be cleaned, transformed, or integrated are not well-defined, resulting in confusion and inconsistencies in dependent workflows.
To address these challenges, you can adopt software engineering best practices in your organization, including the following:
- Data observability measures like data monitoring, alerts, and rollback
- Auditing
- Version control
- Change management
- Data tests
You can use version control tools like Git to track changes to a dataset made by multiple team members who are working on it simultaneously or implement data monitoring to promptly notify team members of pipeline issues that can impact data analysis.
These practices can help ensure that everyone on your team works together effectively, reducing the likelihood of errors or inconsistencies in their work.
Lack of data governance
Gartner analysts estimate that by 2025, 80% of organizations will fail to scale their digital businesses because they have not taken a modern approach to data governance.
Organizations that don’t implement proper data governance measures can cause various problems for data teams, including lack of clear ownership, undiscoverable and duplicated data, access control issues, and unreliable or useless data.
Moreover, without proper data governance, it becomes difficult to locate data assets and identify which datasets are being used for which purposes. This leads to duplicate metrics and business logic, which can be time-consuming and costly.
For instance, if no one is responsible for ensuring the accuracy and completeness of customer data, different departments may use different versions, leading to inconsistencies and poor decision-making. Or, if multiple teams are using different data sources to track customer engagement, it may become difficult to identify the source of any discrepancies in the data.
Access control is another major issue when data governance is lacking. If sensitive data is accessible to everyone in the organization, compliance issues with data privacy regulations can occur.
To address the lack of data governance, you need to establish clear policies, procedures, and standards for data management. You will also need to assign roles and responsibilities for managing data assets. Consider incorporating data governance tools, such as data catalogs, data lineage, and access control systems, to help improve the discoverability and management of your data assets and provide better control over access to sensitive data.
To ensure that your data assets are well-managed and provide value for decision-making, you need to properly embed data governance in your overall data strategy and culture. Educate your employees about the importance of data governance and provide training on best practices.
Why data teams keep repeating the same mistakes
The ongoing struggle data teams have with the same age-old challenges can be attributed to various reasons, including but not limited to the following:
- Lack of reflection and learning: Data teams that don’t prioritize reflection and learning from past mistakes keep repeating the same errors.
- Ineffective collaboration: If team members don’t effectively communicate with each other, important information and feedback can be lost. Mistakes will then be repeated.
- Inconsistent implementation of best practices: Even if teams are aware of best practices, they may not consistently implement them. This can be due to a lack of time, resources, or buy-in from team members.
- Inadequate documentation: It can be challenging to learn from past mistakes and avoid repeating them in the future if data teams don’t document their processes, decisions, and outcomes.
- Failure to adapt: The data landscape is constantly evolving. Teams that don’t adapt their processes to changing circumstances may find themselves repeating the same mistakes over and over again.
Overcome your data problems with the right data solution
While data-driven technologies have modernized the data landscape, data teams still find themselves entangled in the challenges that come with inadequate data management. The following are some of the steps you can take to address these challenges:
- Create shareable data catalogs and lineage that foster communication and collaboration between teams.
- Implement data contracts between teams to ensure compliance with standardized procedures.
- Adopt software engineering best practices, like version control.
- Establish and enforce data governance policies and procedures for access control. Assign roles and responsibilities to promote ownership and accountability.
If you’re concerned about where to start incorporating these solutions in your organization, Y42 has got you covered — from fully managed data pipelining and collaboration in the cloud to secure data governance with data catalogs, contracts, and lineage.
Category
In this article
Share this article